The prize for the best thesis in computer science goes to a physics student
Niklas Maximilian Heim, Master’s student in computational physics at the Niels Bohr Institute, University of Copenhagen, receives this year’s prize for best thesis in computer science. The prize is the annual award from the Danish Society for Computer Science (Dansk Selskab for Datalogi), chosen among nominees from all 8 Danish universities, awarded the year after submission. This year is the first time ever it has been awarded to a physics student.
Looking a year into the future, prediction versus truth: Ocean sea surface heights off the coast of Japan, predicted by the neural network compared to the actual climate simulation data.
In his winning thesis, Automated Anomaly Detection in Chaotic Time Series, Niklas Heim developed new machine-learning methods and software that automatically discover outliers in ocean simulation data. The goal is to detect new oceanic and atmospheric phenomena hidden in the enormous data sets produced by climate simulations, for example shifts in ocean currents or wind patterns. The methods can be used in many other fields where discovering anomalies is key, from financial transactions to biology.
A Neural Network for Physics Simulation Time Series
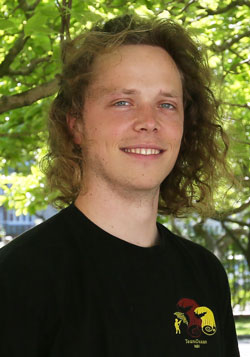
After undergraduate studies with Markus Jochum's Team Ocean, Niklas Heim continued onto NBI's Computational Physics specialization. Heim made good use of what he learned in both lines of study: his thesis with the eScience Group, supervised by Brian Vinter and James Avery, combines methods from artificial intelligence with oceanography to come up with a new type of neural network that can understand turbulent physics simulation time series. Starting from echo state networks, a type of neural networks for predicting chaotic 1D time series, Heim added elements from classical image analysis and geometry, resulting in a system that was strong enough to predict ocean current patterns a year into the future. Comparing to the real data let him quantify the quality of predictions. Then, suddenly worsening predictions indicates an anomalous behaviour has been found.
The oceans – the real as well as the oceans of computer simulations – are unexplored
Like the real oceans, the vast majority of the simulated oceans are unexplored: the simulation data are so colossal in size that no human could look through it all. This makes it crucial to build methods that can automatically analyse these enormous data sets and discover when and where human physicists should look.
Heim’s anomaly-detection software successfully discovered the Kuroshio (Black Tide) sea current bimodality off the coast of Japan without human help. Here is a boundary between fast- and slow flowing water, which is stable over a number of years before suddenly, without warning, rapidly changing into a new shape. Its behavior has serious implications for the sea ecology of the area including fishing and climate. While this is a known phenomenon, discovering it automatically was a breakthrough that opens up for discovering new, unknown ocean phenomena by the same method. Next, the methods will be put to the test in a new collaboration between Team Ocean, eScience, and Niklas Heim to investigate important regions of the oceans.
Sea current predictions is just the beginning
Heim’s methods apply much more broadly than ocean analysis. His software, available as open source, can be applied to many different datasets or computer simulations, and he is now continuing his work on anomaly detection as a Ph.D. fellow at the Artificial Intelligence Center, Czech Technical University. Here, he uses machine learning methods to analyse the dynamics of Tokamak fusion plasma, the fuel of a future fusion reactor: “We focus on learning the unknown governing equations of the plasma. Like this it will be possible to obtain easily interpretable results, which is not the case with the popular machine learning approaches.”
Being able to predict plasma collapse even briefly before it happens, beside saving millions of Euros, would bring us a crucial step closer to making fusion energy reality.