Congratulations to Emy Alerskans
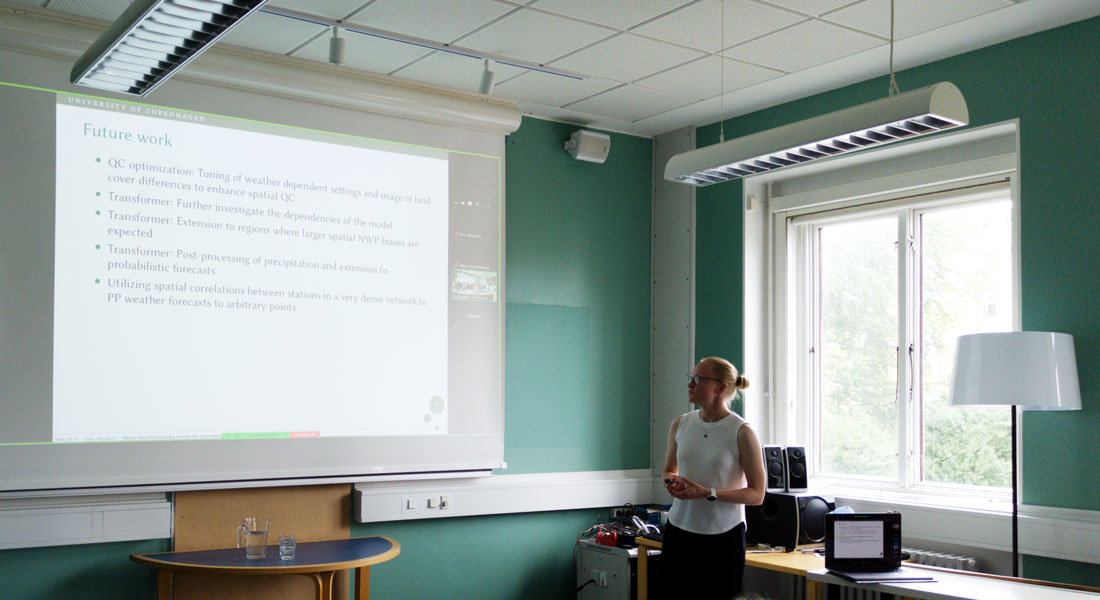
Congratulations to Emy Alerskans at DMI & Physics of Ice, Climate and Earth who successfully defended her PhD thesis on 29 August 2022, and obtained the degree of Doctor of Philosophy.
Title: Hyper-local forecasting system for agricultural applications
Abstract:
Meteorological observations and weather forecasts are important for agricultural applications as the weather has a significant impact on the development and yield of crops, the water and fertilization need and the susceptibility of crops to pests and diseases. Temporally and spatially dense observations and accurate local weather forecasts have the potential to aid short-term agricultural applications, such as e.g. harvesting, irrigation and application of pesticides. This Ph.D. thesis aims at investigating the use of a dense network of private weather stations (PWSs) for agricultural applications, with a focus on producing site-specific forecasts. As data from PWSs often are associated with larger uncertainties than traditional meteorological observations quality control (QC) is important. Here, a framework for tuning spatial QC methods for a dense network of meteorological stations is presented, showing that the performance of the QC method depends on the error magnitude, time of day and station density. In addition, statistical methods for postprocessing 2 m temperature forecasts from a global numerical weather prediction (NWP) model are developed and site-specific forecasts are produced. First, linear and adaptive methods requiring only a short training period are investigated, whereafter the Transformer model, a non-linear machine learning model, is developed. Both models improve upon the raw NWP forecast, with the linear model currently performing best for coastal stations and the Transformer model performing best for inland stations. Furthermore, a similar or even slightly better performance compared to a highresolution NWP model was obtained for certain cases using the Transformer model. The Transformer model is hereafter extended to post-processing of 2 m relative humidity forecasts, for which it outperforms the raw NWP forecast. Moreover, the performance is slightly better or similar to that of a high-resolution NWP model. However, a dependence on distance to the coast is still observed. In addition, PWS 2 m wind speed observations are used to estimate the roughness length and are evaluated for extrapolation to 10 m. These results are further applied as the Transformer model is extended to post-processing of near-surface wind speed forecasts in a preliminary study.
Overall, this study has illustrated the potential of a dense network of PWSs and post-processing to obtain site-specific forecasts for agricultural applications. However, future work is still needed to realize the full potential.
Supervisor: Eigil Kaas
Evaluation Committee: Jens Hesselbjerg Christensen (chair), Irene Schicker, Jens Bremnes